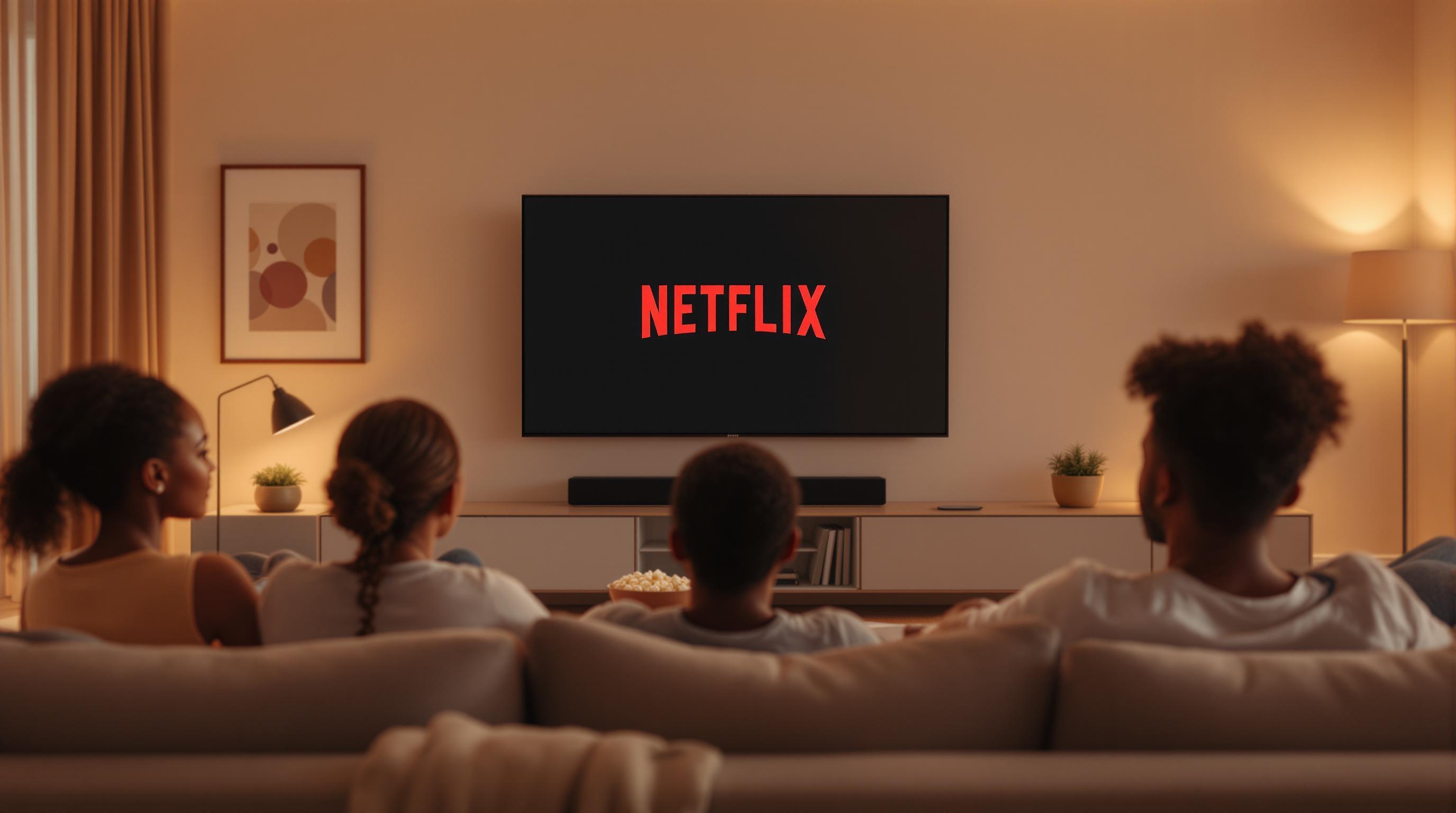
How Netflix Predicts What You Watch
Netflix uses advanced AI to recommend 80% of what you watch. It analyzes billions of data points daily, including:
- Your behavior: Watch time, completion rates, search patterns, and pauses.
- Content details: Over 250 attributes like genre, cinematography, and soundtrack.
- Taste groups: Segments users into 1,000+ groups based on viewing habits.
By combining collaborative and content-based filtering, Netflix creates personalized suggestions within 24 hours. It also adjusts for time, device, and regional trends, ensuring recommendations fit your context. For example, mobile users see shorter content during weekdays, while TV users get 4K options.
Netflix constantly updates its system, using A/B tests and real-time data to refine thumbnails and preferences. With deep learning, it predicts what you'll enjoy next - even before you know it.
Netflix Research: Recommendations
Core Components of Netflix Recommendations
Netflix's recommendation engine uses advanced systems that combine multiple filtering methods and data analysis techniques. The platform processes billions of interactions daily to deliver personalized content suggestions to its massive audience.
Two Main Filtering Methods: Collaborative and Content-Based
Netflix's recommendation system is powered by two key filtering methods that work together. Collaborative filtering focuses on analyzing viewing patterns among users with similar tastes, while content-based filtering evaluates specific features of shows and movies.
Filtering Type | Focus Area | Main Benefit |
---|---|---|
Collaborative | User behavior patterns | Identifies hidden connections |
Content-based | Title attributes & metadata | Ideal for niche preferences |
Hybrid approach | Combines both methods | Boosts accuracy with diverse data |
Combining Filters with Micro-Genres
Netflix uses over 3,000 micro-genres to categorize its content in detail. These "alt-genres" are paired with user behavior data to make precise recommendations, ranging from broad categories to ultra-specific ones like "Emotional Independent Crime Movies" [2].
"Our AI examines 250+ content attributes per title, from cinematography style to soundtrack tempo." - Netflix Chief Product Officer (Variety 2024) [3]
This hybrid approach uncovers subtle viewing patterns. For instance, analyzing 12 million interactions showed a 34% completion rate for the micro-genre "Suspenseful Sci-Fi Thrillers", solidifying its appeal [2][3].
Taste Groups and Viewing Behaviors
Netflix segments its audience into more than 1,000 unique taste groups, built on various factors:
- Viewing habits: Frequency of binge-watching and completion rates
- Genre preferences: Primary and secondary interests
- Engagement patterns: Time spent browsing versus watching
- Content abandonment: Points where viewers stop watching
These groups are constantly updated using real-time data. For example, if viewers in a "Documentary Enthusiasts" group consistently finish investigative series, they'll see more recommendations in that category [2][5].
These detailed groupings play a key role in Netflix's data collection methods, which will be explored in the next section.
Netflix's Data Collection Methods
Netflix uses a detailed tracking system that focuses on real-time viewing habits rather than static preferences. By combining direct user feedback with indirect behavioral signals, the platform gives 10 times more weight to watched content than to thumbs-up or thumbs-down ratings [3][6].
User Ratings vs Watching Behavior
Signal Type | Weight | Key Metrics Tracked |
---|---|---|
Implicit (Behavior) | 10x | Watch time, completion rates, rewatches |
Explicit (Ratings) | 1x | Thumbs up/down, manual preferences |
Behavioral data reveals some interesting trends. For example, users who repeatedly watch sitcoms like The Office log 2.4 times more daily viewing hours compared to those who don't [3].
"The algorithm prioritizes completion rates over ratings - finishing a bad movie says more than rating a good one." - Stratoflow Technical Analysis [4]
Time and Device Influence on Recommendations
Netflix’s recommendation system adjusts based on when and how users watch, tailoring suggestions to both time of day and device type. Here's how it works:
- Time-Based Viewing Patterns
- Device-Specific Adjustments
In a 2024 test, a Stranger Things thumbnail featuring Millie Bobby Brown performed 23% better on mobile devices compared to one showcasing the demon monster [4].
To keep recommendations relevant, Netflix reduces the impact of older data by 20% each month. This approach balances fresh suggestions with established viewing habits [7][6].
sbb-itb-94859ad
AI Systems and Live Updates
Netflix uses real-time AI processing to turn raw data into personalized recommendations. Its system handles over 1 million events per second, leveraging machine learning models to suggest content tailored to individual users.
AI Analysis of Video Content
Netflix's deep learning models dive deep into video content, analyzing multiple elements simultaneously. They evaluate visual aspects like color schemes and camera angles, while also processing audio features such as soundtrack tempo. This creates detailed content profiles that enhance filtering techniques [3].
Content Analysis Type | Elements Analyzed | Purpose |
---|---|---|
Visual Analysis | Scene composition, lighting, cinematography | Align with visual preferences |
Audio Processing | Music tempo, dialogue patterns, sound effects | Capture mood and pacing |
Netflix's Natural Language Processing (NLP) system categorizes content into over 3,000 micro-genres by analyzing reviews and descriptions [6]. This detailed classification helps uncover subtle patterns in user preferences.
"The algorithm prioritizes multi-modal analysis, combining visual, audio, and textual signals to understand content at a deeper level than traditional genre classifications." - Netflix Technology Blog [3]
Testing and Selecting Thumbnails
Netflix uses A/B testing to fine-tune thumbnail selection. The system evaluates different versions by tracking metrics like click-through rates, completion rates, and viewing duration across various demographics [2].
For example, in a 2024 regional test, anime-style thumbnails boosted engagement by 35% in Japan compared to other styles. This led to automated regional adjustments in artwork [8]. Such dynamic optimization complements earlier device-specific adjustments.
Viewing Context and Regional Trends
Netflix's AI adjusts recommendations based on viewing context and cultural influences. It employs a weighted approach that factors in:
During major cultural events, the system temporarily highlights relevant content. For instance, during Brazil's Carnival, local comedy specials are prioritized without disrupting personalization [3]. These cultural adjustments play a key role in reducing subscriber churn, saving Netflix $1 billion annually [1].
Results and Updates to Netflix AI
AI Progress: Past to Present
Netflix's recommendation system has come a long way, evolving into an advanced AI-driven engine that now drives over 80% of content views [4][5].
Here’s a quick look at its key milestones:
Year | Technology Advancement | Impact |
---|---|---|
2013 | Taste communities implementation | Improved group-based recommendations |
2020 | Deep learning integration | Better content analysis |
2024 | Real-time reinforcement learning | Faster, 24-hour adaptation cycles |
Deep learning has been a game-changer for Netflix, allowing for more accurate personalization and quicker adjustments to viewer habits.
Success Rates and Updates
Netflix's AI boasts a 75% completion rate for suggested content [4]. It also maintains a 60% discovery ratio for new recommendations, ensuring users get a mix of familiar and fresh options [7].
To achieve these results, Netflix frequently updates its models:
- Core recommendation models: Updated weekly
- Thumbnail and presentation layers: Refreshed every 48 hours
- Real-time behavioral signals: Adjusted instantly [3][5]
For example, during "The Witcher" Season 3, AI-optimized thumbnails boosted completion rates by 18% [4].
On the technical side, Netflix has slashed recommendation latency to just 300 milliseconds, far better than the industry average of 2 seconds [4]. Their system also adapts to user behavior - morning viewers, for instance, prefer content that's 22% shorter compared to evening viewers [5], enabling smarter, time-sensitive recommendations.
Conclusion: Next Steps for Streaming AI
Netflix's AI is set to focus on three major upgrades between 2024 and 2026, building on its hybrid filtering system [4][3]:
Priority | Netflix Benefit | Timeline |
---|---|---|
Cross-device continuity | Smooth mobile and TV transitions | 2025 |
Mood-based matching | Improved completion rates | Late 2024 |
Custom trailers | Tailored previews | 2026 |
These updates leverage Netflix's expertise in micro-genre categorization and real-time user adaptation. The addition of mood-based matching, in particular, marks a bold step in creating even more personalized content recommendations [3].
Despite these advancements, challenges remain. 37% of users still experience decision fatigue, even with Netflix's advanced recommendation system [4][5]. Multimodal AI integration is expected to address this by refining how content is matched to individual preferences [3].
Experts predict Netflix will explore deeper content features, such as predictive pause points and dynamic story arcs, with prototypes already in testing [3][6].
To maintain quality, Netflix keeps human oversight in the loop, with 12% of suggestions manually adjusted [5]. As viewing habits and regional preferences shift, the platform continues to enhance its contextual understanding to stay ahead.
FAQs
What percentage of Netflix content is influenced by its recommendation algorithm?
Around 80% of the content people watch on Netflix comes from its recommendation system. This powerful tool analyzes viewing habits from millions of subscribers worldwide to suggest what to watch next [1][10].
How does Netflix's personalization algorithm work?
Netflix's personalization algorithm uses a mix of advanced filtering techniques to offer tailored content recommendations. These include:
- Collaborative filtering: Matches users with others who have similar viewing habits.
- Content-based filtering: Matches titles based on attributes like genre, themes, or cast.
- Contextual filtering: Adjusts recommendations based on factors like the device being used or the time of day.
The system also processes thousands of "micro-genres" and detailed content tags to pinpoint patterns that align with your preferences [9].
What powers Netflix's AI recommendations?
Netflix's AI recommendation engine uses real-time data to fine-tune content suggestions. Unlike older demographic-based systems, this AI focuses on viewing behavior - analyzing what you watch and how you interact with the platform. This shift, implemented after 2020, helps Netflix quickly adapt recommendations and reduce subscriber churn [3]. It's a dynamic, behavior-driven system designed to keep viewers engaged, complementing the live update features discussed in the AI Systems section.